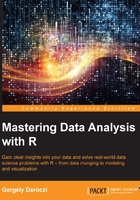
Chapter 2. Getting Data from the Web
It happens pretty often that we want to use data in a project that is not yet available in our databases or on our disks, but can be found on the Internet. In such situations, one option might be to get the IT department or a data engineer at our company to extend our data warehouse to scrape, process, and load the data into our database as shown in the following diagram:

On the other hand, if we have no ETL system (to Extract, Transform, and Load data) or simply just cannot wait a few weeks for the IT department to implement our request, we are on our own. This is pretty standard for the data scientist, as most of the time we are developing prototypes that can be later transformed into products by software developers. To this end, a variety of skills are required in the daily round, including the following topics that we will cover in this chapter:
- Downloading data programmatically from the Web
- Processing XML and JSON formats
- Scraping and parsing data from raw HTML sources
- Interacting with APIs
Although being a data scientist was referred to as the sexiest job of the 21st century (Source: https://hbr.org/2012/10/data-scientist-the-sexiest-job-of-the-21st-century/), most data science tasks have nothing to do with data analysis. Worse, sometimes the job seems to be boring, or the daily routine requires just basic IT skills and no machine learning at all. Hence, I prefer to call this role a data hacker instead of data scientist, which also means that we often have to get our hands dirty.
For instance, scraping and scrubbing data is the least sexy part of the analysis process for sure, but it's one of the most important steps; it is also said, that around 80 percent of data analysis is spent cleaning data. There is no sense in running the most advanced machine learning algorithm on junk data, so be sure to take your time to get useful and tidy data from your sources.
Note
This chapter will also depend on extensive usage of Internet browser debugging tools with some R packages. These include Chrome DevTools
or FireBug
in Firefox. Although the steps to use these tools will be straightforward and also shown on screenshots, it's definitely worth mastering these tools for future usage; therefore, I suggest checking out a few tutorials on these tools if you are into fetching data from online sources. Some starting points are listed in the References section of the Appendix at the end of the book.
For a quick overview and a collection of relevant R packages for scraping data from the Web and to interact with Web services, see the Web Technologies and Services CRAN Task View at http://cran.r-project.org/web/views/WebTechnologies.html.